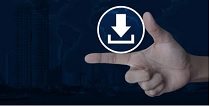
To retain the sign information and thus predict B N instead of | B N|, a secondary so-called polarity model is utilized. However, neural network models do not show clear outperformance compared to the linear regression models. We also calculate the Heidke skill scores for d| B N|/dt by deriving d| B N|/dt from | B N| predictions. The neural network models show significant increases over the linear regression models for | B N| thresholds. The neural networks are then compared against multivariate linear regression models driven with the same inputs at each station using Heidke skill scores with thresholds at the 50, 75, 85, and 99 percentiles for | B N|. Each neural network is driven by solar wind and interplanetary magnetic field inputs from the NASA OMNI database spanning from 2000–2015 and is fine tuned for each station to maximize the effectiveness in predicting | B N|. In this study, we train multi-variate Long-Short Term Memory neural networks to predict magnitude of north-south component of the geomagnetic field (| B N|) at multiple ground magnetometer stations across Alaska provided by the SuperMAG database with a future goal of predicting geomagnetic field disturbances. 6Department of Electronics and Electrical Engineering, University of Bath, Bath, United Kingdomĭuring periods of rapidly changing geomagnetic conditions electric fields form within the Earth’s surface and induce currents known as geomagnetically induced currents (GICs), which interact with unprotected electrical systems our society relies on.4Department of Electrical and Computer Engineering, University of New Hampshire, Durham, NH, United States.3Department of Physics and Astronomy and Space Science Center, University of New Hampshire, Durham, NH, United States.2NASA Goddard Space Flight Center, Greenbelt, MD, United States.1Department of Physics and Geophysical Institute, University of Alaska, Fairbanks, AK, United States.Keesee 3, Victor Pinto 3, Md Shaad Mahmud 4, Chigomezyo Ngwira 5 and Shishir Priyadarshi 6
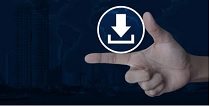